Why preseason projections seem to annually underestimate the Spurs
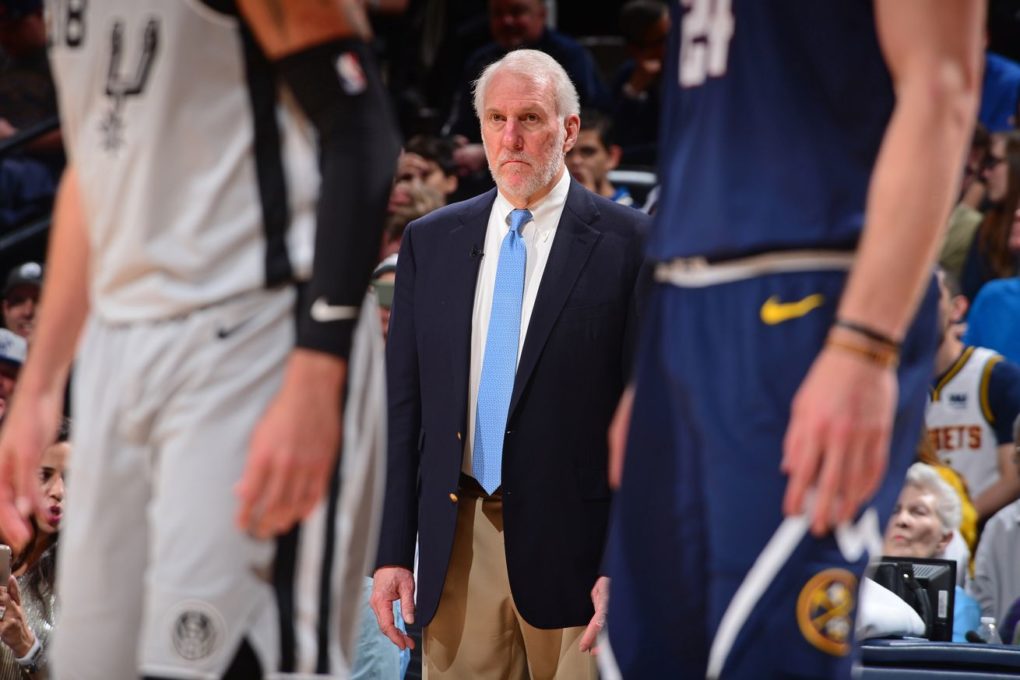
Photo by Bart Young/NBAE via Getty Images
Are the Spurs perennially more than the sum of their projected parts? Data analyst (and Spurs fan) Jacob Goldstein has answers for that question and more.
On Tuesday, polling and analysis website FiveThirtyEight released its Way-Too-Early Projections for next season, which unapologetically had the Spurs predicted to end their 22-year playoff run by winning 37 games in 2019-20, ahead of only the Suns, Grizzlies and Kings in the West. Not that it has to, but the article offers no real explanation for the Spurs’ projection, noting the competition in the conference and making just one (1) direct reference to SA in the entire summary by calling them “fading postseason relics” in passing.
Preseason projections are more about content than science. That’s not a knock on their process or data so much as a note that they’re not meant to serve as a simulation of the regular season. What they do is give something of a barometer of where teams stand based on certain data points and more or less how they might finish, all things being equal and, even better than that, they get us clicking, reacting and (unfortunately) blogging about why we agree or disagree with them.
And here we are.
The Spurs don’t figure to be title contenders next season, and thus whatever corner of the Internet that occasionally writes about them should have no shortage of grounded, nuanced takes that highlight the team’s weaknesses. Yet, the reason that preseason projections such as the above merit some kind of response and exploration is how they speak to a wider Spurs theme that, I believe, many fans buy into: the idea of the Spurs as gestalt, or as an organization that historically comes together to be greater than the sum of its parts.
At face value, there would appear to be some meat to the argument that models underestimate the Spurs. For the 2015-16 and 2016-17 seasons, FiveThirtyEight projected them to win 57 and 52 games, respectively; last October they had them at 37-45. They ended up winning 67, 61, and 48. Even if you include the wacky 2017-18 season that was completely shortcircuited by the Kawhi Saga — one in which the website projected them to win 50 games — the Spurs have outperformed projections by an average of 6.75 wins over the last 4 years. Vegas odds, while steeped in completely different principles, also err on the low side — something that FiveThirtyEight’s own Nate Silver examined in an article aptly called, “Stop Betting Against Gregg Popovich.”
Synonymous with San Antonio’s success over the past two-plus decades, Pop is a key component to this, as are a number of other Spursy intangibles like culture and continuity. These factors are, simultaneously, 1) things we relate disproportionately with San Antonio over most other organizations, 2) capable of helping maximize individual talent, and 3) seemingly impossible to quantify into an algorithm in an attempt to assign them actual value.
To get some inside understanding of what goes into a sophisticated projection model, I reached out to Jacob Goldstein is lead data scientist at BBall Index, and developer of a metric called Player Impact Plus Minus (PIPM). (You can follow him on Twitter at @JacobEGoldstein.) In Goldstein’s own model, the Spurs are projected to win 39.7, which is better than 37 but still 8 wins less than last season’s group. Jacob was nice enough to give detailed answers explaining his model, how it projected the Spurs, and some bigger picture thoughts on some areas where computer projections and real-world performance may not align.
Bruno Passos: Your model currently has the Spurs finishing 39-43 in 2019-20, which is slightly better than FiveThirtyEight’s projection of 37-45 but still lower than what most fans expect coming off of last season’s 48-34 finish.
So, first things first. Why do you hate the Spurs?
Jacob Goldstein: I love this question because I get it pretty often, and not just from Spurs fans. Pretty much anytime someone doesn’t like a projection for their team it’s taken as me disrespecting their franchise. It’s just numbers, there’s never any bad intentions. Especially given I am a Spurs fan, the “wow you just hate the Spurs” comments are most amusing. I find projections a useful way to preview the NBA season, but of course they’re not the be-all-end-all or there would be no joy in even playing the season!
BP: If these are indeed not just arbitrary numbers of pure bias, could you briefly sum up how your model works and/or a handful of the data points it takes into account?
JG: My model is designed to function around my player value metric, Player Impact Plus-Minus. In short, PIPM is an impact metric that combines the boxscore with variance adjusted on/off plus-minus data to estimate a player’s value to their team on the offensive and defensive side of the court per 100 possessions played. Using multiple years of data for added predictive power, I project what a player’s PIPM impact will be next season. Once I have the estimated impact of each player, I use another model to estimate the team’s minutes distribution. Combining the minutes for each player and impact for each player leads to an overall team strength value per 100 possessions. There are a few adjustments I do on these team strength values, such as adjusting for the estimated spacing of a team, but that’s the high level look of how my model and most statistical models work.
BP: In a few lines, could you sum up the biggest factors/assumptions that have the Spurs projecting as a lottery team right now?
JG: The short version is the model sees the rest of the Western Conference as getting better while the Spurs do not. Sure the Spurs did things this summer (bringing back Gay, bringing in Carroll) that the model did like, but everything was balanced out by the stuff the model was not a fan of (signing Lyles, trading Bertans, drafting Samanic). My earliest run of the model put the Spurs around 45 wins, but then the rest of the West outpaced them with moves. Between the star players getting older, the team staying stagnant and the rest of the West improving around them, the model just isn’t impressed with the Spurs’ Summer.
BP: Several models last year had SA finishing remarkably below their eventual 48-34 record, especially after the injury to Dejounte Murray. Historically, it’s seemed like a healthy Spurs team consistently outperforms most models and expectations, including Vegas. Do you agree with the fans’ running assumption that they’re routinely greater than the sum of their parts on paper?
JG: Kind of, which I know is a bit of a cop-out answer. Back when the Spurs were on their 50+ win streak, they were loved by statistical models and consistently projected to continue their regular season dominance and get home-court for the playoffs. I think one area that models have underrated them is the Pop factor, which is just the difficulty in accounting for the impact the greatest coach in NBA history can have lifting up his team. Especially in the last few years when they have not been truly in championship contention, Pop has clearly been able to get the team functioning beyond the sum of their parts. If that will continue is hard to say, but there’s definitely a statistical issue when it comes to measuring the impact a coach can have on players.
BP: If so, is there any way for models to account for such intangibles in projecting a Pop-coached Spurs team? Are Spurs fans stuck in a cycle of being triggered by these yearly projections?
JG: There are a few ways you can account for coaching, but most all of them introduce a significant amount of noise given the difficulty in estimating the value of someone who isn’t even on the court. At BBall Index, we have coaching optimization grades that look at how well a coaching staff gets players to perform compared to what we estimate their talent level as. There’s the possibility that could be introduced to the model to adjust the impact estimate of players coming and leaving from each team according to who the coach is. There have also been attempts at coaching RAPM (essentially estimate a coach’s impact as if he were a 6th player on the court using a fancy regression technique), but it has had mixed results and seems to take a very large sample (as in multiple years) before it stabilizes at all.
BP: Is there any team on the opposite side of this presumptive spectrum that was way better on paper going into last season and underperformed (not as a result of injury)? Adding onto that, is there any team that traditionally underperforms versus what the models project? If so, could you hazard a guess as to what tangible/intangible factors into that trend?
JG: It’s hard to pick teams that really underperformed that much because of how rampant the injuries were. The Wizards fell short, but lost their all-star PG for most of the year. The Timberwolves fell short because of the Butler trade. The Pelicans and Lakers fell short because of, uhm, internal turmoil. The other misses are tanking, which is always impossible to predict. Underperformance is almost always related to injury or tanking.
BP: I mentioned coaching, but there are other — perhaps more anecdotal — factors that people often associate with the Spurs’ way. Those include continuity and culture that — again, perhaps anecdotally — tend to maximize talent. On the flipside, they’re less prone to some of the negative intangibles like tanking and (usually, still true) turmoil. Are such intangibles:
a) too difficult to capture and then plug into a model?
b) not significant enough factors to be worth accounting for in a model?
c) both?
JG: Continuity is definitely something that is captured by models and helps teams. My model includes a regression factor for players changing teams because of the continuity changing impact. Some of those intangibles though are just too tough to capture. You can model tanking, but it would be a factor within a season simulation and not done at the team level because of how hard it is to figure out who will actually tank. I think the maximizing talent thing is real, and I talked about it a bit in my description of our coaching optimization grades. Coach Pop is one of the top coaches in our database at maximizing player impact based off their talent and putting them in situations to succeed.
BP: So, bringing this back to last season, because I think what most fans see is a team that stood pat with a mind to build on a 48-34 group while adding a healthy Murray, seasoned Walker, and continuity that they really missed for the first time in a while. When viewed that way, a 40-or-below win total (essentially an 8+ gap in wins) would appear to insinuate (as you noted) a major drop-off from Aldridge/Gay/DeRozan as well as some fluke-y factors last season that are not likely repeated. Rank these explanations for the 8-win gap:
a) The 2018-19 Spurs overachieved
b) The 2019-20 Spurs are markedly worse (or the competition got markedly better)
c) Missing intangibles (good and bad) that the model doesn’t account for
JG: I would put B first, A second and C third. I think the West is legitimately much harder this year because the elite talent is better spread out across 6-7 teams. The Spurs did overachieve last year, their point differential was closer to that of a 44-45 win team than a 48 win team. Because of the high consistency, I don’t think the “Pop effect” on new players will really have a huge impact outside of what is expected.
BP: Can you identify any X factors/swing players/other variables that would most improve the Spurs’ outlook, from a data perspective?
JG: It’s hard to say who would helps the Spurs at this point because the free agency pickings are very slim. The best remaining free agent is quite possibly Kyle Korver, whose shooting would really be able to help the Spurs. A potential buy-low guy is Dwight Howard if Pop really wants to play two bigs, but there’s a lot of risk along with him too. There just are not many players left who are really able to impact a game. It’s possible the best move at this point is to look to a trade for improvement, moving one of DeRozan or Aldridge for a younger player or good draft picks for the future. The Spurs might be counting on massive internal improvement, with either Dejounte Murray or Derrick White taking an all-star level jump.
BP: If your 39.5 projection is where the Vegas line was drawn for SA, are you taking the over or under? Confidently or tentatively? What about the Vegas line of 43.5?
JG: At 39.7 projected wins, I would take the over with relative confidence. While I personally don’t think this is a super high upside Spurs team, I think there’s enough talent there, and they will be trying the entire season, to be able to beat up on crappy tanking teams come March and April. At 43.5 though, I’d probably take the under. I think they are a half-step below the bottom half of the Western playoff teams without getting a jump from Murray or White.
BP: If I told you the Spurs end up winning 50 games in 2019-20, give me your one-sentence reaction/presumptive explanation about the factor — currently unaccounted for in the model — that most contributed to that.
JG: If you told me they were winning 50 games, I would say the top reason is Dejounte Murray coming back and not just becoming a good offensive player but an elite one. He is already an elite defensive player, but to push the Spurs that much higher he would have to just be the best player on the team on both ends.
Why preseason projections seem to annually underestimate the Spurs
Source: Pounding The Rock